统计与数据科学学院专家报告:庞善起教授学术报告
报告题目:正交表的构造及其应用进展
摘 要:正交表在统计学中主要用于各种试验设计问题,这就意味着它们在人类研究的各个领域都非常重要,正交表的构造被称为21世纪极具挑战性的公开问题。实际应用中,还往往需要具有某些性质的正交表。本报告主要介绍由正交分划方法构造的高强度正交表、Schematic 正交表,以及正交表在量子信息理论中的应用。
报告时间:2024年11月26日14:00-15:00
报告地点:统计与数据科学学院109教室
专家简介:庞善起,现任河南师范大学数学与统计学院二级教授,博士生导师,校学术委员会委员,统计学一级学科博士点学科带头人,中国现场统计研究会常务理事,中国现场统计研究会多元分析应用专业委员会副理事长,中国科协科技人才奖项评审专家,河南省密码学重点学科带头人。主要从事试验设计的理论和应用研究,我们构造的大量新的正交表特别是混合正交表出现在南开大学和美国ATT和SAS网站上。已在Annals of Statistics,Nature子刊 npj Quantum Information, Statistica Sinica,Information Sciences, Quantum Science and Technology等SCI及国内外期刊上发表论文90余篇,一些研究成果被美国著名统计学家 Hedayat,Sloane,Stufken的专著:Orthogonal Arrays: Theory and Application,Springer,1999全文引用。主持国家自然科学基金面上项目5项。
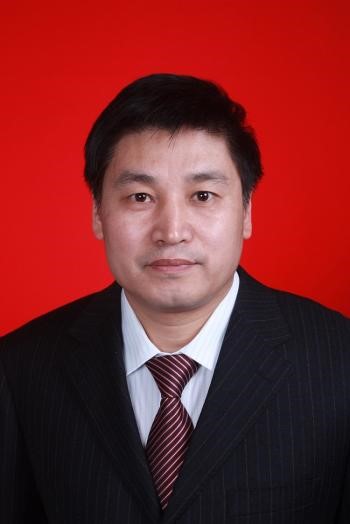
统计与数据科学学院专家报告:何煦副研究员学术报告
报告题目:A distance metric-based uniform subsampling method for nonparametric models
摘 要:Taking subset samples from the original data set is an efficient and popular strategy to handle massive data that is too large to be directly modeled. While in most theoretical advances the subset samples are collected independently with equal probability, for accurate inference and prediction it is beneficial to employ a fast subsampling scheme to select observations intelligently. The majority of existing subset selecting methods are provided for linear models or their extensions. In this paper, we propose a novel subsampling method for efficient nonparametric modeling of high-volume data sets. It is a proportionate sampling method that uses distance metric-based strata to select subsamples. To minimize the maximal distance from pairs of samples that locate in the same stratum, Voronoi cells of thinnest covering lattices are used to partition the space. With the help of an algorithm to quickly identify the cell an observation locates in, the computational cost of our subsampling method is proportional to the number of observations and irrelevant to the number of cells, which makes our method applicable to extremely large data sets. Results from simulated studies and real data analysis show that the new method is remarkably better than existing approaches when used in conjunction with $k$-nearest neighbor or Gaussian process models.
报告时间:2024年11月26日15:00-16:00
报告地点:统计与数据科学学院109教室
专家简介:何煦,本科毕业于北京大学数学科学学院,博士毕业于威斯康星大学麦迪逊分校统计系。2012年博士毕业后入职中国科学院数学与系统科学研究院系统科学研究所,现任副研究员。主要研究方向包括实验设计,特别是计算机仿真实验的设计及分析。在Annals of Statistics,Journal of the American Statistical Association,Biometrika,Journal of Machine Learning Research等杂志发表论文十余篇,曾主持国家自然科学基金委优秀青年基金、面上项目、青年基金。
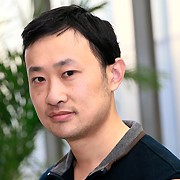
统计与数据科学学院专家报告:王兆军教授学术报告
报告题目:Semi-supervised distribution learning
摘 要:This study addresses the challenge of distribution estimation and inference in a semi-supervised setting. In contrast to prior research focusing on parameter inference, this work explores the complexities of semi-supervised distribution estimation, particularly the uniformity problem inherent in functional processes. To tackle this issue, we introduce a versatile framework designed to extract valuable information from unlabeled data by approximating a conditional distribution on covariates. The proposed estimator is derived from the K-fold cross-fitting strategy, exhibiting both consistency and asymptotic Gaussian process properties. Under mild conditions, the proposed estimator outperforms the empirical cumulative distribution function in terms of asymptotic efficiency. Several applications of the methodology are given, including parameter inference and goodness-of-fit tests.
报告时间:2024年11月26日16:00-17:00
报告地点:统计与数据科学学院109教室
专家简介:王兆军,南开大学统计与数据科学学院执行院长、教授,教育部“长江学者奖励计划”特聘教授,享受国务院政府特殊津贴专家。兼任国务院学位委员会统计学科评议组成员,全国统计教材编审委员会委员,中国工业与应用数学学会副理事长,中国统计教育学会副会长,中国工业统计教学研究会副会长,中国概率统计学会副理事长。曾任国家统计专家咨询委员会委员、中国现场统计研究会副理事长、天津市现场统计研究会理事长,天津工业与应用数学学会理事长。获全国百篇优博指导教师、教育部自然科学二等奖及天津市自然科学一等奖。
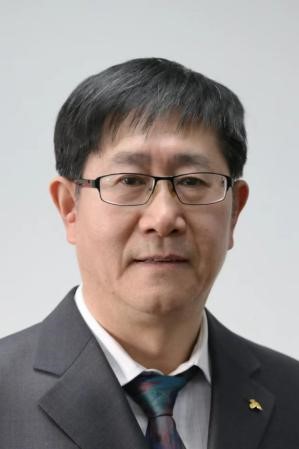